RECAST: Interactive Auditing of Automatic Toxicity Detection Models
Austin P. Wright, Omar Shaikh, Haekyu Park, Will Epperson, Muhammed Ahmed, Stephane Pinel, Diyi Yang, Duen Horng (Polo) Chau
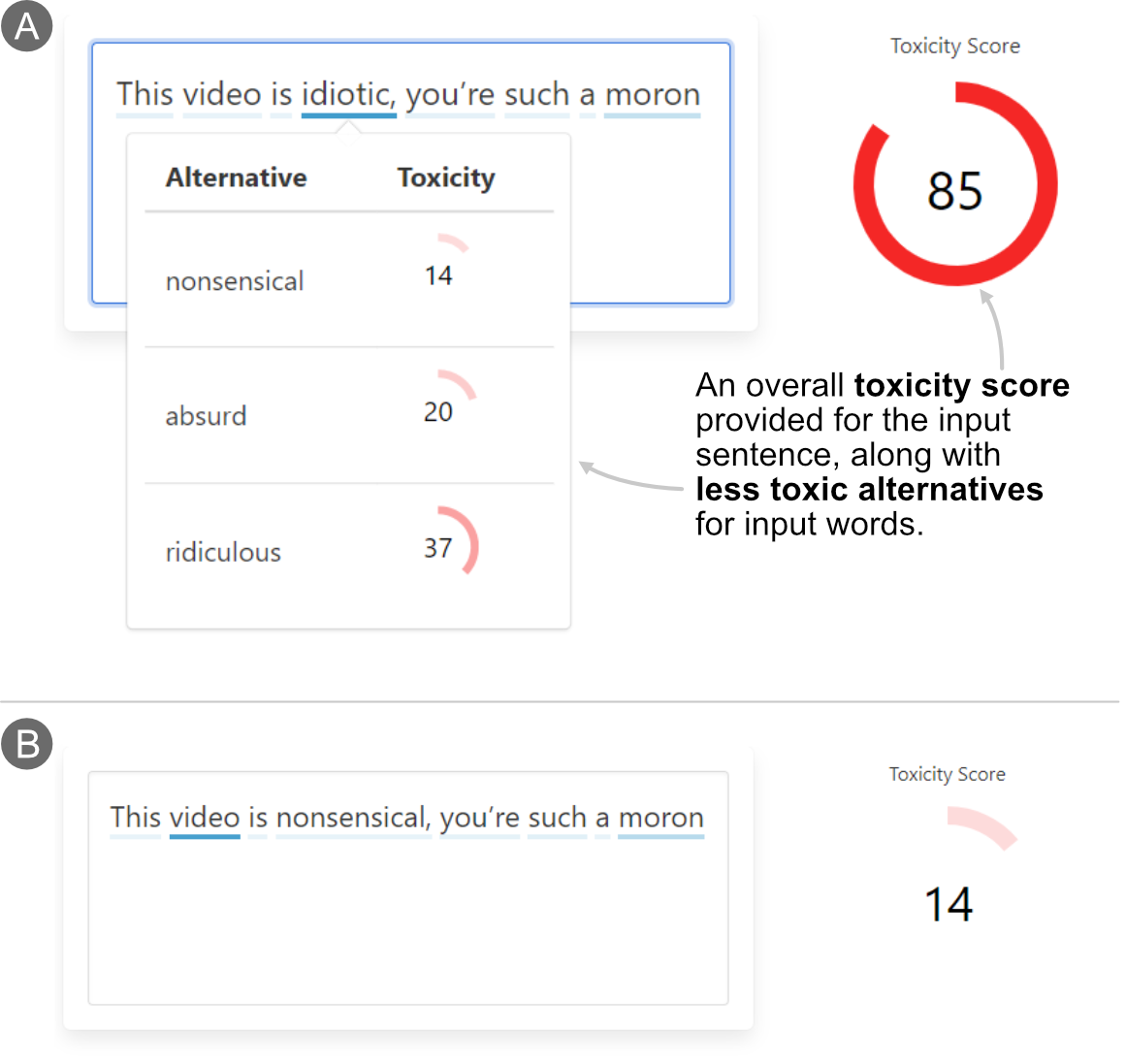
Abstract
As toxic language becomes nearly pervasive online, there has been increasing interest in leveraging the advancements in natural language processing (NLP) to automatically detect and remove toxic comments. Despite fairness concerns and limited interpretability, there is currently little work for auditing these systems in particular for end users. We present our ongoing work, RECAST, an interactive tool for auditing toxicity detection models by visualizing explanations for predictions and providing alternative wordings for detected toxic speech. RECAST displays the attention of toxicity detection models on user input, and provides an intuitive system for rewording impactful language within a comment with less toxic alternative words close in embedding space. Finally we propose a larger user study of RECAST, with promising preliminary results, to validate it’s effectiveness and useability with end users.
Citation
RECAST: Interactive Auditing of Automatic Toxicity Detection Models
Austin P. Wright,
Omar Shaikh,
Haekyu Park,
Will Epperson,
Muhammed Ahmed,
Stephane Pinel,
Diyi Yang,
Duen Horng (Polo) Chau
Interactive Auditing of Automatic Toxicity Detection Models
24th ACM Conference on Computer-Supported Cooperative Work & Social Computing. 2021.
Project
PDF